In April 2018 a new video of Barack Obama surfaced on the Internet. Against a backdrop that included both the American and presidential flags, it looked like many of his previous speeches. Wearing a crisp white shirt and dark suit, Obama faced the camera and punctuated his words with outstretched hands: “President Trump is a total and complete dipshit.”
Without cracking a smile, he continued. “Now, you see, I would never say these things. At least not in a public address. But someone else would.” The view shifted to a split screen, revealing the actor Jordan Peele. Obama hadn’t said anything—it was a real recording of an Obama address blended with Peele’s impersonation. Side by side, the message continued as Peele, like a digital ventriloquist, put more words in the former president’s mouth.
In this era of fake news, the video was a public service announcement produced by BuzzFeed News, showcasing an application of new artificial-intelligence (AI) technology that could do for audio and video what Photoshop has done for digital images: allow for the manipulation of reality.
The results are still fairly unsophisticated. Listen and watch closely, and Obama’s voice is a bit nasally. For brief flashes, his mouth—fused with Peele’s—floats off-center. But this rapidly evolving technology, which is intended for Hollywood film editors and video game makers, has the imaginations of some national security experts and media scholars running dark. The next generation of these tools may make it possible to create convincing fakes from scratch—not by warping existing footage, as in the Obama address, but by orchestrating scenarios that never happened at all.
The consequences for public knowledge and discourse could be profound. Imagine, for instance, the impact on the upcoming elections if a fake video smeared a politician during a tight race. Or attacked a CEO the night before a public offering. A group could stage a terrorist attack and fool news outlets into covering it, sparking knee-jerk retribution. Even if a viral video is later proved to be fake, will the public still believe it was true anyway? And perhaps most troubling: What if the very idea of pervasive fakes makes us stop believing much of what we see and hear—including the stuff that is real?
Many technologists acknowledge the potential for sweeping misuse of this technology. But while they fixate on “sexy solutions for detection and disclosure, they spend very little time figuring out whether any of that actually has an effect on people’s beliefs on the validity of fake video,” says Nate Persily, a law professor at Stanford University. Persily studies, among other topics, how the Internet affects democracy, and he is among a growing group of researchers who argue that curbing viral disinformation cannot be done through technical fixes alone. It will require input from psychologists, social scientists and media experts to help tease out how the technology will land in the real world.
“We’ve got to do this now,” Persily says, “because at the moment the technologists—necessarily—drive the discussion” on what may be possible with AI-generated video. Already our trust in democratic institutions such as government and journalism is ebbing. With social media a dominant distribution channel for information, it is even easier today for fake-news makers to exploit us. And with no cohesive strategy in place to confront an increasingly sophisticated technology, our fragile collective trust is even more at risk.
Innocuous Beginnings
The path to fake video traces back to the 1960s, when computer-generated imagery was first conceived. In the 1980s these special effects went mainstream, and ever since, movie lovers have watched the technology evolve from science-fiction flicks to Forrest Gump shaking hands with John F. Kennedy in 1994 to the revival of Peter Cushing and Carrie Fisher in Rogue One. The goal has always been to “create a digital world where any storytelling could be possible,” says Hao Li, an associate professor of computer science at the University of Southern California and CEO of Pinscreen, an augmented-reality start-up. “How can we create something that appears real, but everything is actually virtual?”
Early on, most graphics came from artists, who used computers to create three-dimensional models and then hand-painted textures and other details—a tedious process that did not scale up. About 20 years ago some computer-vision researchers started thinking of graphics differently: rather than spending time on individual models, why not teach computers to create from data? In 1997 scientists at the Interval Research Corporation in Palo Alto, Calif., developed Video Rewrite, which sliced up existing footage and reconfigured it. The researchers made a clip of JFK saying, “I never met Forrest Gump.” Soon after, scientists at the Max Planck Institute for Biological Cybernetics in Tübingen, Germany, taught a computer to pull features from a data set of 200 three-dimensional scans of human faces to make a new face.
The biggest recent jump in the relationship among computer vision, data and automation arguably came in 2012, with advances in a type of AI called deep learning. Unlike the work from the late 1990s, which used static data and never improved, deep learning adapts and gets better. This technique reduces objects, such as a face, to bits of data, says Xiaochang Li, now in the department of communication at Stanford. “This is the moment where engineers say: we are no longer going to model things,” she says. “We are going to model our ignorance of things and just run the data to understand patterns.”
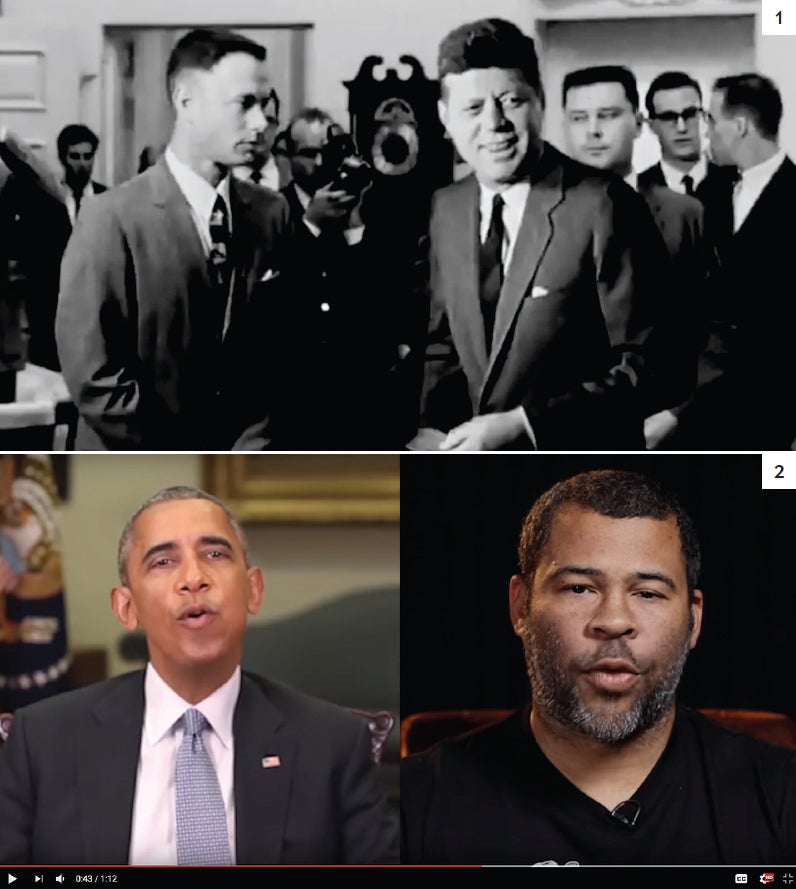
Deep learning uses layers of simple mathematical formulas called neural networks, which get better at a task over time. For example, computer scientists can teach a deep-learning tool to recognize human faces by feeding it hundreds or thousands of photographs and essentially saying, each time, this is a face or this is not a face. Eventually when the tool encounters a new person, it will recognize patterns that make up human features and say, statistically speaking, this is also a face.
Next came the ability to concoct faces that looked like real people, using deep-learning tools known as generative networks. The same logic applies: computer scientists train the networks on hundreds or thousands of images. But this time the network follows the patterns it gleaned from the examples to make a new face. Some companies are now using the same approach with audio. In 2018 Google unveiled Duplex, an AI assistant based on software called WaveNet, which can make phone calls and sounds like a real person—complete with verbal tics such as uhs and hmms. In the future, a fake video of a politician may not need to rely on impersonations from actors like Peele. In April 2017 Lyrebird, a Canadian start-up, released sample audio that sounded creepily like Obama, Trump and Hillary Clinton.
But generative networks need big data sets for training, and that can require significant human labor. The next step in improving virtual content was to teach the AI to train itself. In 2014 researchers at the University of Montreal did this with a generative adversarial network, or GAN, which puts two neural networks in conversation. The first is a generator, which makes fake images, and the second is a discriminator, which learns to distinguish between real and fake. With little to no human supervision, the networks train one another through competition—the discriminator nudges the generator to make increasingly realistic fakes, while the generator keeps trying to trick the discriminator. GANs can craft all sorts of stuff. At the University of California, Berkeley, scientists built one that can turn images of horses into zebras or transform Impressionist paintings by the likes of Monet into crisp, photorealistic scenes.
Then, in May 2018, researchers at the Max Planck Institute for Informatics in Saarbrücken, Germany, and their colleagues revealed “deep video,” which uses a type of GAN. It allows an actor to control the mouth, eyes and facial movements of someone else in prerecorded footage. Deep video currently only works in a portrait setup, where a person looks directly at the camera. If the actor moves too much, the resulting video has noticeable digital artifacts such as blurred pixels around the face.
GANs are not yet capable of building complex scenes in video that are indistinguishable from ones captured in real footage. Sometimes GANs produce oddities, such as a person with an eyeball growing out of his or her forehead. In February 2018, however, researchers at the company NVIDIA figured out a way to get GANs to make incredibly high-resolution faces by starting the training on relatively small photographs and then building up the resolution step by step. And Hao Li’s team at the University of Southern California has used GANs to make realistic skin, teeth and mouths, all of which are notoriously difficult to digitally reconstruct.
None of these technologies are easy for nonexperts to use well. But BuzzFeed’s experiment hints at our possible future. The video came from free software called FakeApp—which used deep learning, though not GAN. The resulting videos are dubbed deepfakes, a mash-up of “deep learning” and “fake,” named after a user on the Web site Reddit, who, along with others, was an early adopter and used the tech to swap celebrities’ faces into porn. Since then, amateurs across the Web have used FakeApp to make countless videos—most of them relatively harmless pranks, such as adding actor Nicolas Cage to a bunch of movies he was not in or morphing Trump’s face onto the body of German chancellor Angela Merkel. More ominous are the implications. Now that the technology is democratized, anyone with a computer can hypothetically use it.
Conditions for Fake News
Experts have long worried that computer-enabled editing would ruin reality. Back in 2000, an article in MIT Technology Review about products such as Video Rewrite warned that “seeing is no longer believing” and that an image “on the evening news could well be a fake—a fabrication of fast new video-manipulation technology.” Twenty years later fake videos don’t seem to be flooding news shows. For one thing, it is still hard to produce a really good one. It took 56 hours for BuzzFeed to make the Obama clip with help from a professional video editor.
The way we consume information, however, has changed. Today only about half of American adults watch the news on television, whereas two thirds get at least some news via social media, according to the Pew Research Center. The Internet has allowed for a proliferation of media outlets that cater to niche audiences—including hyperpartisan Web sites that intentionally stoke anger, unimpeded by traditional journalistic standards. The Internet rewards viral content that we are able to share faster than ever before, Persily says. And the glitches in fake video are less discernible on a tiny mobile screen than a living-room TV.
The question now is what will happen if a deepfake with significant social or political implications goes viral. With such a new, barely studied frontier, the short answer is that we do not know, says Julie Carpenter, a research fellow with the Ethics + Emerging Sciences Group, based at California State Polytechnic University, San Luis Obispo, who studies human-robot interaction. It is possible we will find out soon enough, with key elections coming up this fall in the U.S., as well as internationally.
We have already witnessed the fallout when connectivity and disinformation collide. Fake news—fabricated text stories designed to look like legitimate news reports and to go viral—was a much discussed feature of the 2016 U.S. presidential election. According to collaborative research from Princeton University, Dartmouth College and the University of Exeter in England, roughly one in four Americans visited a fake news site during the five weeks between October 7 and November 14, 2016, mostly through the conduit of their Facebook feeds. Moreover, 2016 marked a low point in the public’s trust in journalism. By one estimate, just 51 percent of Democrats and 14 percent of Republicans said they trusted mass media.
The science on written fake news is limited. But some research suggests that seeing false information just once is sufficient to make it seem plausible later on, says Gordon Pennycook, an assistant professor of organizational behavior at the University of Regina in Saskatchewan. It is not clear why, but it may be thanks to “fluency,” he says, or “the ease at which it is processed.” If we hear Obama call Trump a curse word and then later encounter another false instance where Obama calls Trump obscene names, we may be primed to think it is real because it is familiar.
According to a study from the Massachusetts Institute of Technology that tracked 126,000 stories on Twitter between 2006 and 2017, we are also more likely to share fake news than real news—and especially fake political stories, which spread further and quicker than those about money, natural disasters or terrorism. The paper suggested that people crave novelty. Fake news in general plays to our emotions and personal identity, enticing us to react before we have had a chance to process the information and decide if it is worth spreading. The more that content surprises, scares or enrages us, the more we seem to share it.
There are troubling clues that video may be especially effective at stoking fear. “When you process information visually, you believe that this thing is closer to you in terms of space, time or social group,” says Elinor Amit, an assistant professor of cognitive, linguistic and psychological sciences at Brown University, whose work teases out the differences in how we relate to text and images. She hypothesizes that this distinction is evolutionary—our visual development came before written language, and we rely more on our senses to detect immediate danger.
Fake video has, in fact, already struck political campaigns. In July 2018 Allie Beth Stuckey, a TV host at Conservative Review, posted on Facebook an interview with Alexandria Ocasio-Cortez, a Democratic congressional nominee from New York City. The video was not a deepfake but an old-fashioned splice of a real interview with new questions to make Ocasio-Cortez appear to flub her answers. Depending on your political persuasion, the video was either a smear job or, as Stuckey later called it in her defense, satire. Either way, it had 3.4 million views within a week and more than 5,000 comments. Some viewers seemed to think Ocasio-Cortez had bombed a real interview. “Omg! She doesn’t know what and how to answer,” one wrote. “She is stupid.”
That all of this is worrying is part of the problem. Our dark ruminations may actually be worse for society than the videos themselves. Politicians could sow doubt when their real misdeeds are caught on tape by claiming they were faked, for example. Knowing that convincing fakes are even possible might erode our trust in all media, says Raymond J. Pingree, an associate professor in mass communications at Louisiana State University. Pingree studies how confident people are in their ability to evaluate what is real and what is not and how that affects their willingness to participate in the political process. When individuals lose that confidence, they are more likely to fall for liars and crooks, he says, and “it can make people stop wanting to seek the truth.”
A Game of Cat and Mouse
To a computer scientist, the solution to a bug is often just more computer science. Although the bugs in question here are far more complex than bad coding, there is a sense in the community that algorithms could be built to flag the fakes.
“There is certainly technical progress that can be made against the problem,” says R. David Edelman of M.I.T.’s Internet Policy Research Initiative. Edelman, who served as a tech adviser under Obama, has been impressed by faked videos of the former president. “I know the guy. I wrote speeches for him. I couldn’t tell the difference between the real and fake video,” he says. But while he could be fooled, Edelman says, an algorithm might pick up on the “telltale tics and digital signatures” that are invisible to the human eye.
So far the fixes fall within two categories. One proves that a video is real by embedding digital signatures, analogous to the intricate seals, holograms and other features that currency printers use to thwart counterfeiters. Every digital camera would have a unique signature, which, theoretically, would be tough to copy.
The second strategy is to automatically flag fake videos with detectors. Arguably the most significant push for such a detector is a program from the Defense Advanced Research Projects Agency called Media Forensics, or MediFor. It kicked off in 2015, not long after a Russian news channel aired fake satellite images of a Ukrainian fighter jet shooting at Malaysia Airlines Flight 17. Later, a team of international investigators pegged the flight’s downing on a Russian missile. The satellite images were not made with deep learning, but DARPA saw the coming revolution and wanted to find a way to fight it, says David Doermann, MediFor’s former program manager.
MediFor is taking three broad approaches, which can be automated with deep learning. The first examines a video’s digital fingerprint for anomalies. The second ensures a video follows the laws of physics, such as sunlight falling the way it would in the real world. And the third checks for external data, such as the weather on the day it was allegedly filmed. DARPA plans to unify these detectors into a single tool, which will give a point score on the likelihood that a video is fake.
These strategies could cut down on the volume of fakes, but it will still be a game of cat and mouse, with forgers imitating digital watermarks or building deep-learning tools to trick the detectors. “We will not win this game,” says Alexei Efros, a professor of computer science and electrical engineering at U.C. Berkeley, who is collaborating with MediFor. “It’s just that we will make it harder and harder for the bad guys to play it.”
And anyway, these tools are still decades away, says Hany Farid, a professor of computer science at Dartmouth College. As fake video continues to improve, the only existing technical solution is to rely on digital forensics experts like Farid. “There’s just literally a handful of people in the world you can talk to about this,” he says. “I’m one of them. I don’t scale to the Internet.”
Saving Reality
Even if each of us can ultimately use detectors to parse the Internet, there will always be a lag between lies and truth. That is one reason why halting the spread of fake video is a challenge for the social media industry. “This is as much a distribution problem as it is a creation problem,” Edelman says. “If a deepfake falls in the forest, no one hears it unless Twitter and Facebook amplify it.”
When it comes to curbing viral disinformation, it is not clear what the legal obligations are for social media companies or whether the industry can be regulated without trampling free speech. Facebook CEO Mark Zuckerberg finally admitted that his platform has played a role in spreading fake news—although it took more than 10 months following the 2016 election. Facebook, after all, was designed to keep users consuming and spreading content, prioritizing what is popular over what is true. With more than two billion active monthly users, it is a tinderbox for anyone who wants to spark an enraging fake story.
Since then, Zuckerberg has promised to act. He is putting some of the burden on users by asking them to rank the trustworthiness of news sources (a move that some see as shirking responsibility) and plans to use AI to flag disinformation. The company has been tight-lipped on the details. Some computer scientists are skeptical about the AI angle, including Farid, who says the promises are “spectacularly naïve.” Few independent scientists have been able to study how fake news spreads on Facebook because much of the relevant data has been on lockdown.
Still, all the algorithms and data in the world will not save us from disinformation campaigns if the researchers building fake-video technology do not grapple with how their products will be used and abused after they leave the lab. “This is my plea,” Persily says, “that the hard scientists who do this work have to be paired up with the psychologists and the political scientists and the communication specialists—who have been working on these issues for a while.” That kind of collaboration has been rare.
In March 2018, however, the Finnish Center for Artificial Intelligence announced a program that will invite psychologists, philosophers, ethicists and others to help AI researchers to grasp the broader social implications of their work. A month later Persily, along with Gary King, a political scientist at Harvard University, launched the Social Data Initiative. The project will, for the first time, allow social scientists to access Facebook data to study the spread of disinformation.
With a responsibility vacuum at the top, the onus of rooting out fake videos is falling on journalists and citizen sleuths. Near the end of the deepfake video of Obama and Peele, both men say: “Moving forward, we need to be more vigilant with what we trust from the Internet. It’s a time when we need to rely on trusted news sources.” It may have been a fake, but it was true.